What is Data Science?
Let’s break the term into its composite parts – data and science. Science works fundamentally through the formulation of hypotheses – educated guesses that seek to explain how something works – and then finding enough reasonable evidence through observations in the real world to either prove the hypothesis right, or falsify it. Data, on the other hand, refers simply to numbers and statistics which we gather for the sake of analysis.
By combining these two, we get data science. What exactly does it mean? Data science is an umbrella term for all techniques and methods that we use to analyze massive amounts of data with the purpose of extracting knowledge from them.
Example of Data Science
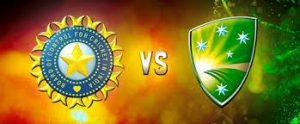
Source : Acadgild
Let’s say you are crazy about Cricket, which I am sure you areJ, and there is an ongoing series between India and Australia. India loses the first two matches, much to your disappointment, and you are eager to know what will happen in the next game. You go online to check the results of past encounters between the two nations and notice a trend – every time India has lost two games in a row against Australia, they have come back strongly in the third. You are convinced India will win the next game, and predict the outcome. To everyone’s surprise, your prediction turns out right. Congratulations, you’re a data scientist!
The numbers and statistics that a data scientist observes in the real world may not be so simple, and he/she might even require software to recognize the underlying trends. Nonetheless, the basic idea is the same. Due to its efficiency in predicting outcomes, data science is useful in developing artificial intelligence – what we will explore next.
Artificial Intelligence:
Artificial intelligence (AI) is a very broad term. Chiefly, it is an attempt to make computers think like human beings. Any technique, code or algorithm that enables machines to develop, mimic, and demonstrate human cognitive abilities or behaviors falls under this category. An artificial intelligence system can be as simple as a software that plays chess, or more complex like a car without a driver. No matter how complex the system, artificial intelligence is only in its nascent stages. We are in what many call “the era of weak AI”. In the future we might enter the era of strong AI, when computers and machines can replicate anything and everything humans do.
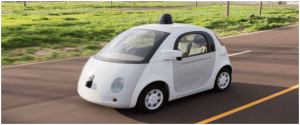
Source : Business Insider
The saying, “be careful what you wish for” is extremely apt when discussing artificial intelligence. The emergence of AI has thrown up new challenges almost as a by-product of the advanced technology. While it’s good that a car which doesn’t need a driver can take you to your destination with little or no effort on your part, it would be terrible if it crashed along the way. Similarly, it’s good that robots can help with work in industries. However, it won’t be so good, if they declared war against humans. I digress. These are discussions for another time, and I want to move on to the topic of machine learning in the next section.
Machine Learning:
Machine learning is one of the hottest technologies right now. It refers to, as the name suggests, a computer’s ability to learn from a set of data, and adapt itself without being programmed to do so. It’s one kind of artificial intelligence that builds algorithms which can analyze input data to predict an output that falls within an acceptable range.
Supervised Learning
Machine learning algorithms can be of different kinds. The most common of these are “supervised algorithms”. These algorithms make observations using “labels”, which are nothing but predictable outcomes.
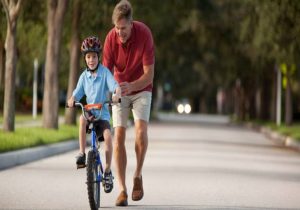
Source : Acadgild
Example
You collect a bunch of questions along with their answers. Using these, you train your model to create a question-answer software. This software responds with an appropriate answer every time you ask it a question. Cortana and other digital assistants, which are essentially speech automated systems in mobile phones or laptops, work this way. They train themselves to work with your inputs and then deliver amazing results according to their training.
Unsupervised Learning
“Unsupervised algorithms” are of a different kind. Unlike its counterpart (supervised algorithms), these algorithms do not have a sample data set to guide their learning or help them predict outcomes. Clustering algorithms are good examples of unsupervised machine learning.
Examples
Let us suppose you’re in a new environment – it’s your first day in college, and you don’t know anyone. You don’t know the people, the culture, where your classes are, nothing. It takes time for you to recognize and classify things you encounter in your college life. You identify the friends in your group, your competition either in class or other activities, the good food items in the canteen, etc. You learn about these things without knowing what to expect and gather information in a haphazard manner. Your method of learning is unsupervised.
Similarly, when NASA discovers new objects in space from time to time, it is a difficult task to classify them. If the object is very different from known objects such as meteoroids or asteroids, then it requires more time and effort to study the object and gather information pertaining to it. With time and more information on the characteristics of the object, it NASA can classify it – either group it with existing objects or classify it as something new altogether. In this scenario, NASA uses an unsupervised learning technique, much like the way unsupervised algorithms function, without predictable outcomes.
I hope you’re beginning to realize that artificial intelligence and machine learning are not quite the same. AI is the science that seeks to help machines mimic human cognition and behavior, while machine learning refers to those algorithms that make machines think for themselves. Simply put, machine learning enables artificial intelligence. That is to say, a clever program that can replicate human behavior is AI. But if the machine does not automatically learn this behavior, it is not machine learning.
Deep Learning
Deep learning is only a subset of machine learning. It is one of the most popular forms of machine learning algorithms that anyone can come across. They use artificial neural networks (ANNs). Artificial neural networks are a family of models similar to biological neural networks in humans. These networks are capable of processing large volumes of data. Neurons (different nodes in the model) are inter-connected and can communicate with each other. Final outputs depend on the weight of different neurons. Essentially, it’s an algorithm that can receive and calculate large volumes of input data, and still churn out meaningful output. In deep learning, the artificial neural network is made up of neurons in different layers. These layers lie one over the other. They are separate and multiple instead of one.
What separates deep learning from other forms of algorithms is its ability to automatically extract features from input data. Unlike feature engineering, it does not require any manual support. It is similar to image recognition processes that do not require particular features in the image for interpretation. The processes up information on the go, and use it to deliver their output. Let us consider an example to understand this.
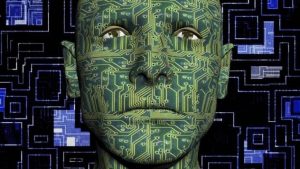
Source : Acadgild
Example
Say you are playing a game along with four of your friends. Your friends are the different layers of the artificial neural network – all standing in a row one behind the other. There is a moderator to enforce the rules of the game. A picture is shown to the first person. His objective is to extract as much information as he can, and pass it on along the row. The game shall go on until the last person can describe the image accurately.While playing, all members standing in queue will have to alter the information, little at a time, to improve the eventual description. Deep learning works in a similar fashion. It refines the information it collects over time to deliver finer results.
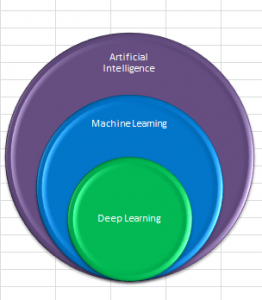
Source : Acadgild
Evolution of AI
The picture above clearly depicts the relationship between artificial intelligence, machine learning, and deep learning. It’s apparent that artificial intelligence is the broadest term. Both machine learning and deep learning are subsets of it. In fact, deep learning is also a subset of machine learning. AI is the broadest term out of the three. It also happens to be the oldest. It originated in the 1950’s when the “Turing Test” became popular. A lot of time and energy was devoted in those days to try and create an algorithm that could mimic human beings. The first real success in this endeavour was achieved when machine learning was introduced in the 70’s.
Machine learning was path-breaking at the time, but it had its limitations. Human curiosity knows no bounds, and the will to create a more robust algorithm persevered. Now, the goal became to overcome the limitations of machine learning.
Fast-forward to 2010 when Google launched its ‘Brain Project’, and the perception of artificial intelligence – of what it meant and could achieve – was changed forever.
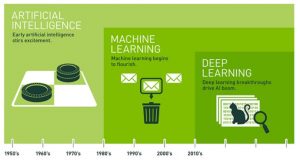
Source : Blogs.nvidia
How Does Data Science Relate to AI, ML & DL?
As mentioned before, data science is an inter-disciplinary field that requires skills and concepts used in disciplines such as statistics, machine learning, visualization, etc. According to Josh wills, a data scientist “is a person who is better at statistics than any software engineer and better at software engineering than any statistician”. He/she is the jack of all trades manipulating and playing with data. How is the data scientist and his field of study related to artificial intelligence, machine learning, and deep learning?
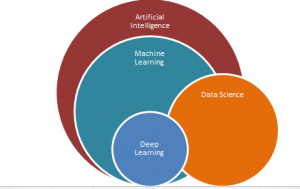
Source : Acadgild
To answer simply, data science is a fairly general term for processes and methods that analyze and manipulate data. It enables artificial intelligence to find meaning and appropriate information from large volumes of data with greater speed and efficiency. Data science makes it possible for us to use data to make key decisions not just in business, but also increasingly in science, technology, and even politics.
Conclusion
Artificial intelligence is a computer program that is capable of being smart. It can mimic human thinking or behavior. Machine learning falls under artificial intelligence. That is to say, all machine learning is artificial intelligence, but not all artificial intelligence is machine learning. For example, a simple scheduling system may be artificial intelligence, but not necessarily a machine that can learn. Then we have deep learning, which is, again, a subset of machine learning. Both – machine learning and deep learning – fall under the broad category of artificial intelligence. Lastly, data science is a very general term for processes that extract knowledge or insights from data in its various forms. Although it has no direct relation to artificial intelligence, machine learning or deep learning, it can be useful to each of the three.